Data Cleansing
Clean customer data is key to efficient processes around your customers.
Data cleansing is an important part of any data quality initiative. Initially, in first time right or in data maintenance. Incorrect or inconsistent data causes a multitude of problems in operational and strategic customer management.
Contact us
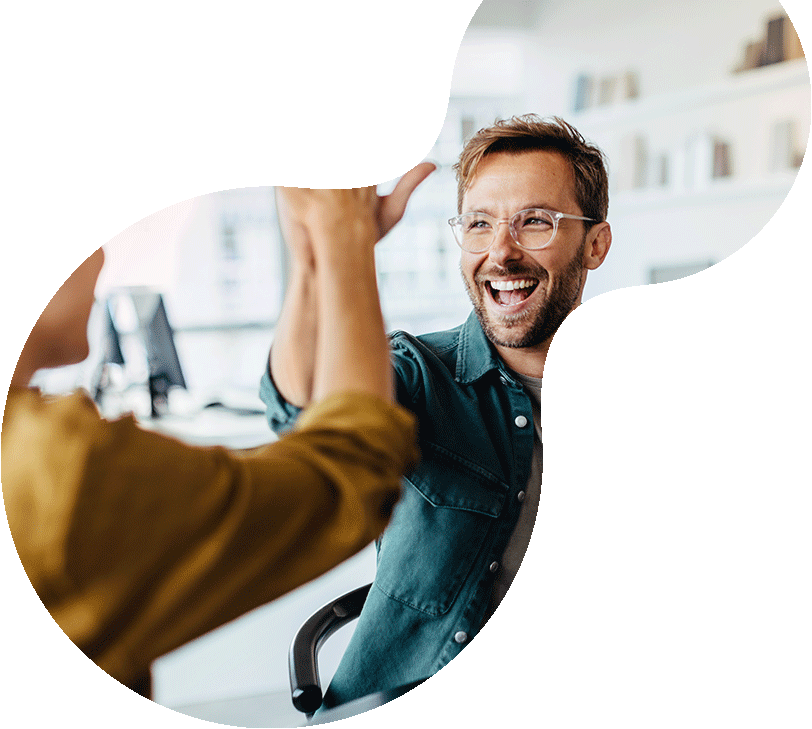
Data cleansing plays an important role in many scenarios: in the cleansing of legacy systems or of master data, in migration projects, in risk management or when it comes to compliance. Cleansed data is also of great importance in customer retention or in dialogue and direct marketing. Cleaned data means everything for success. Or the other way around: without cleansed data, everything is nothing! Because: garbage in, garbage out!
When is data cleansing important?
Although customer data is critical to success in business, it is often neglected and at best insufficiently, but certainly not adequately, observed and maintained. This results in inadequate data quality, which in turn has far-reaching negative consequences, such as inefficient processes, increased costs, lost revenue and annoyed or even lost customers. Signs of insufficient data quality are:
- Important contact information of prospects and customers is incorrect or missing.
- The same customer is created multiple times in the system or information is distributed across multiple systems.
- Employees spend a lot of time searching for customer information.
- High volume of returns after mailings.
Different scenarios of data cleansing
Data cleansing is not a 'one-off' action
Not all data cleansing is the same. This is because the phase you are in with your data and its quality determines when data cleansing should take place in your process in order to achieve the desired result. The respective focus of the measures can also vary. The scenarios are:
Initial data cleansing. Your entry into clean data.
During initial data cleansing, your entire dataset is checked and, as far as possible, automatically cleansed. Errors that cannot be corrected automatically are marked so that they can be cleaned up by the users or your data steward. Duplicates should be consolidated as a so-called Golden Record. If you have many different data sources and/or if your data comes from different countries, your initial data cleansing activities will be a bit more complex. However, your gain in the end is an up-to-date, complete, correct, and unique data set that you can work well with immediately.
Ideally, you will have prepared for the initial data cleansing with a Data Assessment. In such an initial analysis, your data is examined for the central quality criteria for data - up-to-dateness, completeness, correctness and overlap. This is the ideal basis for initial cleansing. Because you already know in advance where the shoe really pinches. And you can plan your quality improvement measures in a targeted manner right from the start.
Typical components of an initial data cleanup are:
- The data is put into a unified format.
- The name components are analyzed.
- A check of the addresses is performed.
- Relocations are updated.
- Addresses are enriched with additional information.
- Duplicates are identified and 'Golden Records' are created.
First Time Right. Clean data from the start.
Data sets are not static collections of customer data, they are alive. There are constant changes, for example, new data records are added or existing ones are supplemented. That's why after the initial cleanup comes the Data Quality Firewall. It prevents initially cleared data sets from becoming creepily contaminated by dynamic data traffic, since incorrect, incomplete or duplicate data successively re-enters the system.
This is the real-time scenario of data cleansing. Nothing enters the system unchecked. In concrete terms, this means that when a new data record is entered into the system, it is first checked to see whether it already exists and whether it is correct at all. This is done with a fully automated, highly precise, error-tolerant duplicate recognition system. If it is not clear whether it is a duplicate or not, the DQ Firewall can display possible alternatives. In a call center or service environment, this is an invaluable advantage.
Errors and contamination are often flushed into the system during data entry. Especially when multiple departments are involved that have different demands and expectations of the data, or the end customers themselves are involved in the entry process. Spelling errors, typos, missing letters or whole words, hearing errors, and typo abbreviations often cause problems when new data is entered or changes are made. By consistently implementing data quality checks directly at the points where data enters the system, the "creeping" contamination of data can be prevented.
Advantages First Time Right:
- Correct customer master data from the very beginning - no matter from which source the data comes or who entered the data - is the most cost-effective way to ensure data quality.
- High-quality data enables efficient processes, right from the start. Subsequent corrections to the data are cost-intensive and are avoided.
- With some mistakes, for example in marketing, there is no second chance for the first bad impression. Mistakes cannot be corrected after the fact. This can be avoided with First Time Right.
- You manage customer master data with at least the same attention as other valuable assets of the company.
Data Maintenance. Preventing data from aging.
Despite all the precautions taken, it makes sense to check and clean up a database 'periodically' within certain periods of time. Unfortunately, data is subject to a daily aging process. This must be counteracted by suitable data quality measures. For example, addresses, telephone numbers and e-mail addresses should be checked regularly for accuracy. This is the only way to determine whether errors have crept in due to external changes or internal changes made to the data. It is also important to regularly check whether data records have been created twice by mistake, for example, in order to ensure the quality of customer data in the long term.
The 1-10-100-rule
„It takes $1 to verify a record as it´s entered, $10 to cleanse and de-dupe it and $100 if nothing is done, as the ramifications of the mistakes are felt over and over again.“
Quelle: SiriusDecisions/Forrester
Reasons for aging data:
- Relocations: Every day, an average of 25,000 people move in Germany. However, not all of them inform their new address, such as banks, insurance companies and other business partners. Without a current address, however, customer contact is lost, for example for sending important mail.
- Renaming: Every year, 45,000 street names and 2,000 place names change in Germany, due to incorporations, renaming or new development areas. These changes must also be continuously tracked in databases, otherwise the correct address will no longer be available.
- Duplicates: They are like a 'wrong fuff' and turn up sooner or later, despite all the care taken. It is therefore advisable to periodically search the entire database for duplicate and multiple data records and then consolidate them so that no valuable information is lost.
You might also be interested in: