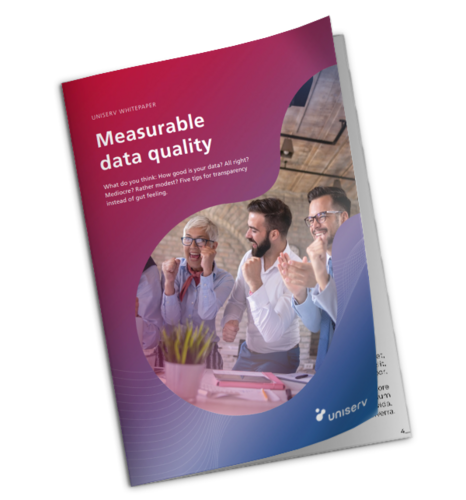
WHITEPAPER
Measurable data quality. 5 tips for more transparency
There's often nothing more than a vague gut feeling when it comes to the quality of your own data. So how do you make it measurable?
Companies collect vast amounts of data via their many, predominantly digital touchpoints. Most of the time, this data remains unused. And then? The question of its quality quickly arises. When evaluating the data quality, the gut feeling often dominates; objective, number- and fact-based transparency is missing!
With the Uniserv whitepaper 'Measurable Data Quality', you will learn how to change from gut feeling to transparency in terms of data quality:
- How complete is your data?
- How many duplicates are included?
- How up-to-date is your data?
- Can you draw analytical results from your data?
- Can you segment your data cleanly?
Download the Uniserv whitepaper 'Measurable Data Quality' now. And put it to the test. Let's be honest - do you have anything to lose? No, only to gain, namely transparency and data 'fit for use!
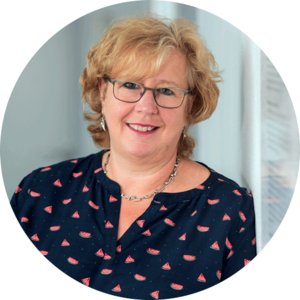
We are here for you.
Our Customer Data Experts will quickly and competently answer your questions and find a suitable solution for your concern