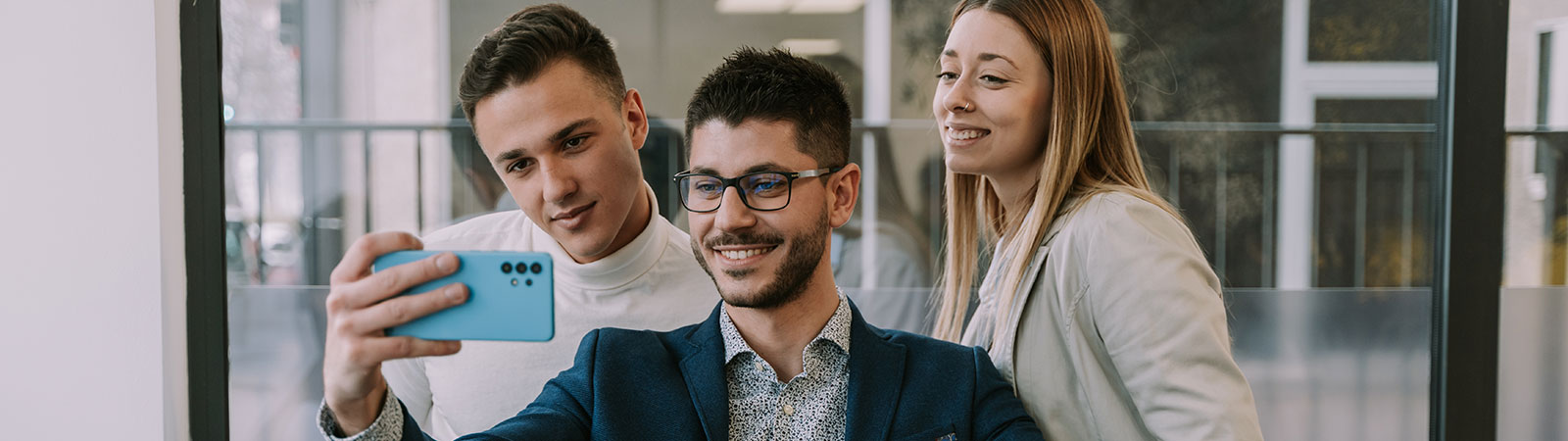
Knowledge
Advantage through knowledge
In our 'Magazine', we provide you with the latest news and background information on all topics relating to data quality. Sometimes short and concise, sometimes more detailed. In the 'Webinars & Events' section, we look forward to exchanging ideas with you, live on site or virtually. aAnd in the 'Whitepapers' section, we go into depth on many different topics. Read on!
Magazine
Get in-depth knowledge and the latest news on data quality - for the successful management of customer data & master data.
Webinars & Events
Whether live on site or virtually - we look forward to an exciting exchange with you and your participation in our webinars & events.
Papers
We share our in-depth knowledge of data quality and customer data management with you. Browse now & download a free paper.