Data Governance
Secure the quality and performance of your customer data on a permanent organizational basis.
Business processes and customer data are highly dynamic and complex. The more digitization comes into focus and the more important data becomes, the more relevant are the considerations of how to make processes more efficient with customer data.
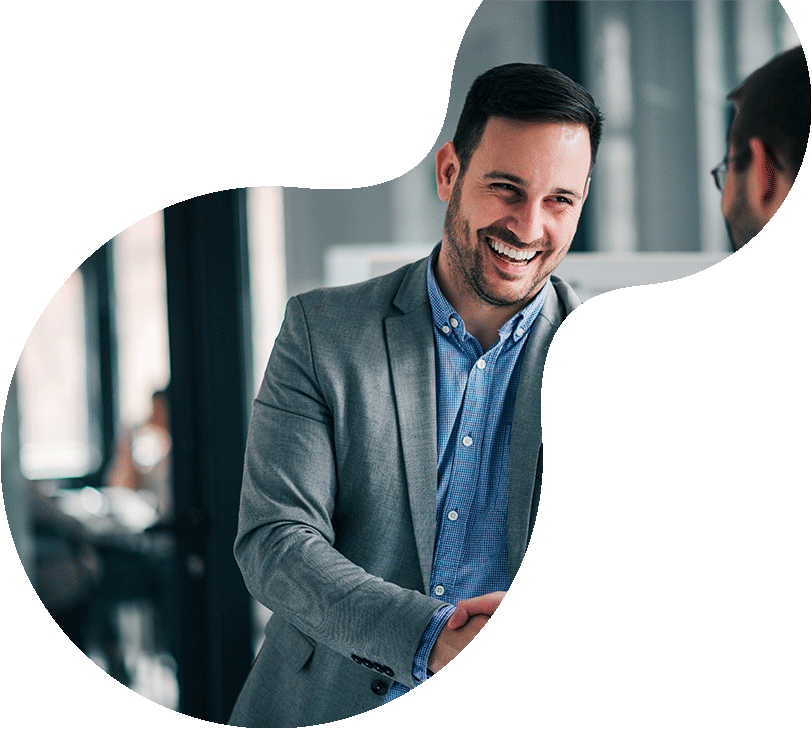
But is the data even ready for use? Does every employee in the company have the data they need? Is compliance liveable or just gray theory? Mastering data operationally is one thing. It is quite another to activate strategic aspects as value creation potentials throughout the company. Only those who can put their data in the right context can handle it profitably. This requires intelligent customer data governance. As a new order. And from the Customer Data Expert: Uniserv!
Develop a data strategy that suits you
We support you in this
With Customer Data Governance made by Uniserv, you define cross-functional and cross-departmental responsibilities and decision-making powers. You enable your company as a 'living organism' to secure and optimize the value-adding customer data processes end-to-end qualitatively and legally. The Uniserv Data Governance Framework spans the umbrella for this. In this respect, customer data governance is not simply a project, but part of the corporate culture. Even more: Customer Data Governance shapes the corporate culture. Our consulting services include in particular:
- process optimization through optimization of the system and process architecture.
- data standardization through optimization of data models and data architecture.
- implementation by defining roles and responsibilities.
- planning the transition by planning the cleansing and migration steps.
Data Governance + Uniserv = Customer Data Governance
Our origin, our 'DNA' so to speak, is to improve the quality of customer data in your systems. This improved data quality leads to resilient business processes. The solutions required for this come from Uniserv itself. With a tailwind from more than 50 years of company history and hundreds of projects. At the same time, we support and accompany you in customer data governance with consulting, technology and services from a single source in the development of your individual data governance framework: with the 'Customer Data Governance Playbook' from Uniserv.
Uniserv Customer Data Governance ensures quality where it is needed in your company. We do not make things more scientific, nor do we complicate, but simplify. We plan your moves so that everyone involved knows what they need for what. And what they can build on, what they can rely on, and above all: a firmly defined time frame on which to rely.
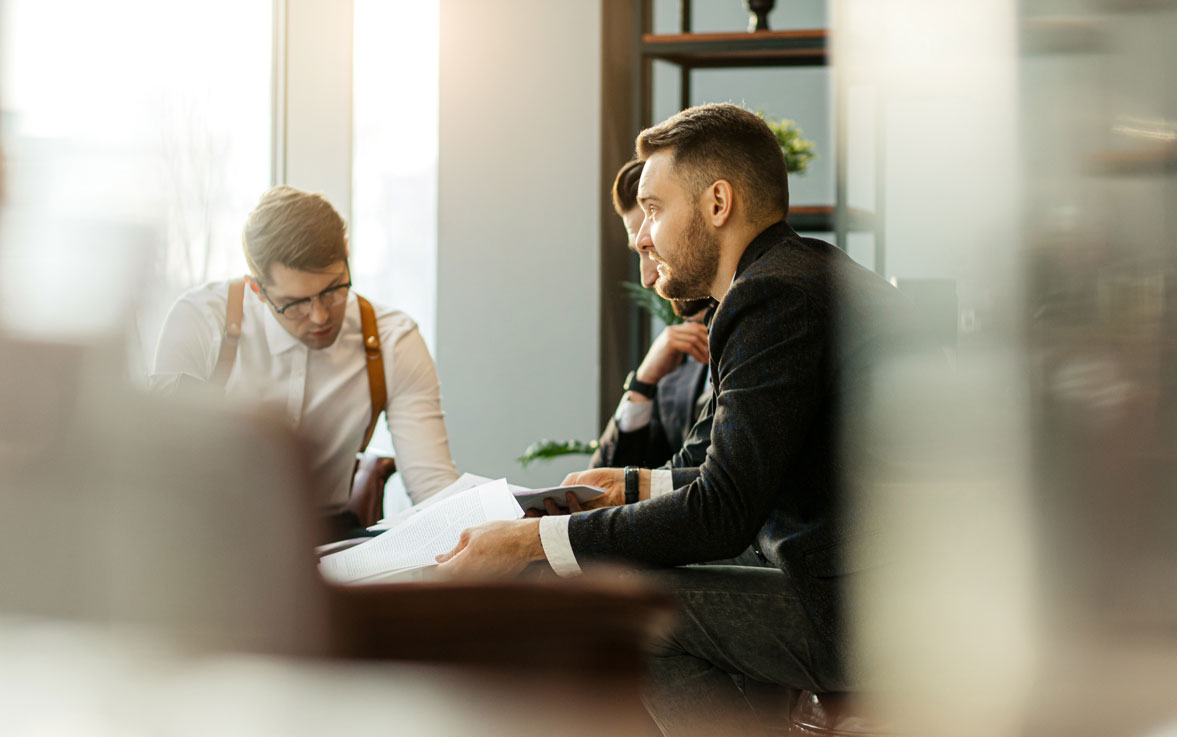
The Uniserv procedure model
How to get to your gata governance framework
The Uniserv approach model for customer data governance is the quintessence of more than half a century of passion for data. The objective is to guide you quickly and purposefully to your individual data governance framework over a defined period of time. For the activation of your strategic value creation from your data. Uniserv is your coach, the partner at your side, with whom you design the development and introduction of the data governance framework. According to your wishes, according to your specifications, according to your individual circumstances. In the end, you will have reduced complexity in terms of processes and data while ensuring future viability: You will know which processes are important, which data you need for them and in what quality, and who must be able to work with them. Reliable, permanent, transparent. The Uniserv process model consists of six packages which build on each other. These can be processed successively or selectively. Which procedure is recommended depends on your specific situation. There is no "one size fits all" here.

Focus.
In an initial assessment, we help you identify your most important customer data value creation processes end-to-end, which on the one hand make the greatest economic contribution to your company and on the other hand map all important internal company processes and thus data interfaces. This is the ACTUAL analysis. Against this, we set the TARGET analysis to develop the delta as a field of action.

Data Accountability.
We advise you on which roles and responsibilities are necessary around your most important identified customer data value creation processes in order to permanently transfer them into an independent organization. Together with you, we now also cover the data level in addition to the business processes in package 1. Here, too, the ACTUAL and the TARGET are ultimately opposed to each other, with a clear delta as the field of action.

Data Standardization.
Data originates through various heterogeneous input channels and is then used by many different departments for different purposes. We help your data managers standardize the respective common data, for maximum permeability and automation, i.e. towards uniform standards for data and data quality. This then naturally also includes a uniform data format.

Master Data Management.
Master data is stored in a large number of applications and databases. Different departments get involved, different stakeholders have demands on the data, and input and maintenance sometimes take place at different points. We support you in ensuring that the master data is available in a uniform and consolidated form at the end of the day and that its quality is permanently assured.

Data Quality.
Once we have created your future data governance framework, we help you identify existing data quality issues, address their root causes, and implement corrective mechanisms for the future. Classically, transparently and traceably. Our goal is to provide you with the highest quality results in the best performance. Simply Customer Data Experts.

Performance Measurement.
Already during the development of your Data Governance Framework you will achieve first performance improvements. We help you to make these transparent and to create long-term KPIs and an action plan to continuously expand your data competence as an organization. After all, once you have achieved something, you don't want to lose it again or put it at risk.
Your advantages in a nutshell
This is what your individual customer data governance framework does for you
- You know your value-adding customer data processes in detail.
- The necessary roles are named and activated in line with your organization.
- Your individual customer data standard model is designed.
- You have a defined set of rules that will permanently ensure master data quality in the future.
- You know the status quo of the quality of your data and how to design the processes of cleansing, enrichment, etc.
- You have suitable software solutions at hand to accompany the entire transformation on the tool side and to implement MDM permanently.
You might also be interested in: