Introducing systems for CRM or marketing automation with data quality in mind
![[Translate to Englisch:] crm-evaluierunge_header.jpg](/fileadmin/content/Wissen/Magazin/Teaser-Headerbilder/crm-evaluierunge_header.jpg)
Companies spend a lot of time on the introduction of a new CRM or marketing automation system. The most common mistake: the horse was " bridled from behind "! Poor quality data and missing processes slow down the new system. For success, the right sequence is crucial: data-process-solution!
High data quality as a final
The hope that the introduction of a new software solution - marketing automation for marketing, CRM for sales - is the starting point for "customers at the push of a button" proves to be a misguided approach in many cases. The prerequisite for success in marketing and sales in the age of digitization is to aggregate the contact data available in the company across platforms and systems into a golden record that enables a 360-degree view of the customer across departments. Only then is a company in a position to make valid and sustainable statements for the conception and implementation of customer-oriented marketing and sales campaigns.
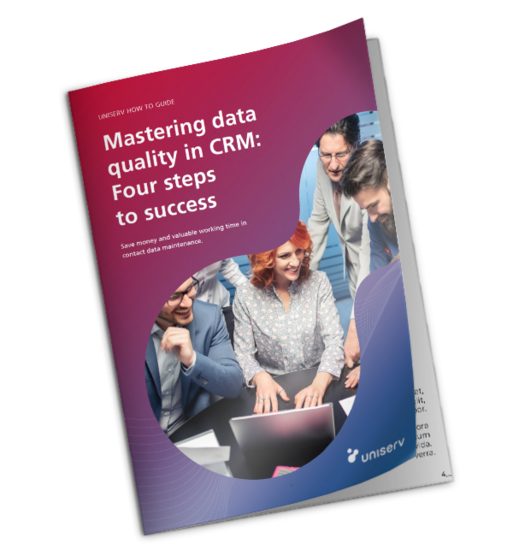
Mastering data quality in CRM: Four steps to success
Save money and valuable working time in contact data maintenance!
The Uniserv How-To Guide shows you transparently and comprehensibly how you can achieve high data quality in four steps and transform your CRM into a real favourite system.
Concrete goals firmly in view
If companies want to strengthen customer acquisition and retention, they usually pursue these three goals when introducing a new CRM or marketing automation solution:
- Increase sales
- Reduce costs
- Reduce risks
In this context, marketing and sales managers want the proverbial "customer at the push of a button". They would like to see these specific pain points solved:
- - Creating a central contact/customer database with a contact/customer profile that is as comprehensive as possible
- - Overview of all relationships/connections with/to the contact/customer
- - Ability to capture/assess the lead/customer potential of the contact
- - Convert contacts into customers
With today's CRM and marketing automation systems, the vision of "customers at the push of a button" seems to be achievable. This is especially true for industries and business models where personal customer contact is no longer necessary at all to close a deal (e-commerce, digital information products, e-learning, cloud software, and many more).
Wrong sequenz
However, many companies do not achieve the desired performance after implementation. They make the mistake of worrying about the software solution first and then about data and processes. This is problematic because data
- is often scattered in silos and therefore incomplete,
- ages due to e.g. relocations, deaths, place and street renaming
- have been created incorrectly or twice or even more frequently due to spelling, reading, listening and typing errors.
A software solution and the analyses created with IT are only as good as the quality of the data processed in these systems
Those who work with unchecked data in the new system will not get out of trouble, according to the motto "garbage in, garbage out". The consequences are:
- Unreliable customer-related processes.
- High return rates after mailings and campaigns.
- Lack of evaluation options for customers and prospects.
- Inaccurate analysis results.
- Less trustworthy recommendations for action.
It's like trying to avoid driving off the road in thick fog. A difficult undertaking. Maybe you are lucky and reach your destination. But sometimes you end up in a ditch.
First the data, then the process, then the solution
If the introduction of a new CRM or marketing automation solution is on the agenda for marketing and/or sales, the focus should first be on the data that will later be worked with. This data must be 'fit for use' in the context of the customer at the push of a button, i.e. it must have the appropriate quality required for the respective process and thus also the application purpose. The data-carrying processes and later the selection of the desired system are based on this.
The right quality means that the data fields that are needed are available. In CRM and marketing automation, for example, this is the e-mail address of a customer or prospect, but also the address, contact data, order history, and so on.
In addition to the necessary data fields, there must also be clarity regarding key dimensions of data quality:
- Completeness
- Accuracy
- Timeliness
- Uniqueness
A data assessment ensures transparency. The data can then be initially cleansed in a data cleansing process. In this way, quality is established in the four dimensions. Data from different sources must be consolidated into a holistic data set, the golden record.
Only when the data and then the processes have been optimized should a company think about the software solutions it uses
If the data is qualitatively optimized and thus trustworthy, one can think in detail about the individual customer processes, such as:
- Lead process in marketing automation
- Nurture routes and data flows
- Offer process in the CRM system
- Steps and checks of new customers in the CRM system
- Dashboarding in CRM
Reliable data makes the difference
In order to provide your (potential) customers with tailored information and offers at the right time and in the right place via the right channel on the right device in the long term, more and more companies are turning to incorporating the wealth of available customer data into corresponding business intelligence analyses in order to gain insights for the future orientation of their business processes and to launch new marketing and sales campaigns on this basis. The results of these campaigns flow into the business intelligence analysis and also form the basis for strategic business decisions. Similar process chains are used in other business areas such as IT, logistics or finance.
When you consider that predictive analytics analyses are usually based on just ten percent of the historical data available in the company, it quickly becomes clear what this means for the quality of this data. Only if the data is carefully maintained over the long term, complete, accurate and up-to-date, will the forecasts be correct. And only if the forecasts are correct will the further adjustments in the processes or the planning of the campaigns as well as the process development in CRM work.
After data cleansing is befor data cleansing
Data quality is not a one-time initiative or action. Customer data needs constant attention and care, data maintenance. It ages, for example, due to moves, deaths, marriages, street and town renamings, or incorporations.
CRM and marketing automation are not systems hermetically sealed off from the environment. Rather, there is a constant exchange of data. Data is deleted, new data is added, and it may even be necessary to search for existing customer data.
A data quality firewall ensures that these system breakthroughs do not lead to creeping contamination of data that has once been optimized to such a high standard. It ensures that only clean, reliable data enters the system from the time of data entry (first time right), and that no data records are created twice or more than once.
First the data, then the process, then the software solution: the formula for success in marketing and sales
Predictive analytics forecasts also depend on the quality of the data used. Only when the data and then the processes have been optimized, i.e. coordinated with each other (fit for use), should a company think about the software solutions used and, if necessary, evaluate possible alternatives to the applications currently available in the company. In this way - and only in this way - is it ensured that the revenue side of the company is also optimally supported, in terms of increasing sales, reducing costs and minimizing risks.
You might also be interested in: