Data Quality Hub
Correct customer data right from the start
With the Data Quality Hub, you ensure that only correct data enters your systems. You also ensure that your data is automatically kept up to date with anti-aging functions or automatically enriched with additional information if required.
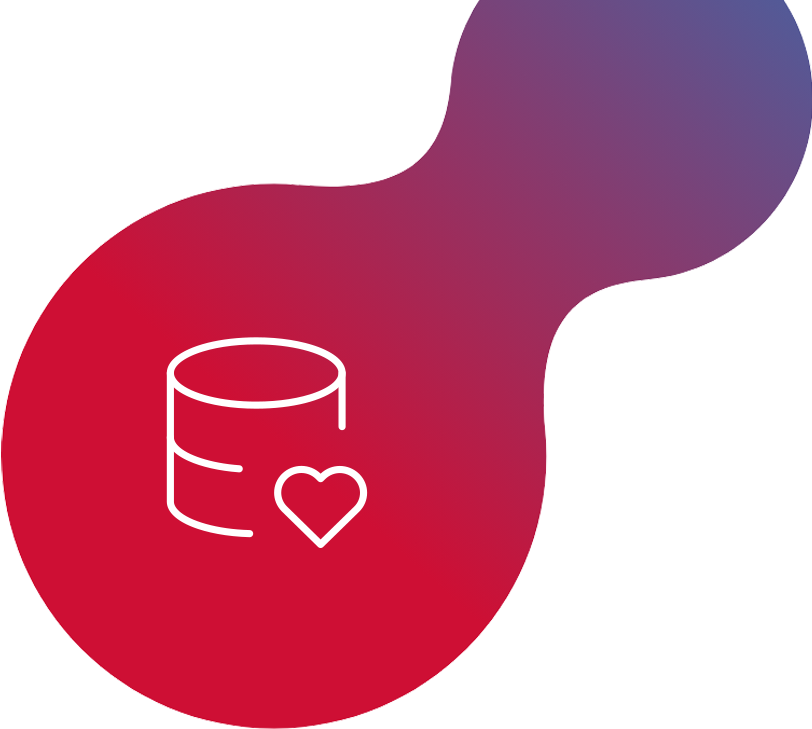
Optimize your customer data along the data quality lifecycle
To get the best out of your data, you need to understand, maintain, protect and monitor it. And you need to do this throughout the entire data quality lifecycle. For these tasks, we offer you a combination of powerful software and experienced consultants.
The Uniserv Data Quality Hub meets your requirements precisely. This ensures that your data always meets the requirements of your business.
Read the success story to find out how we mastered the challenges of data quality together with R+V Versicherung.
What does the Data Quality Hub do?
The Data Quality Hub offers a unique combination of flexibility, efficiency and user-friendliness. Unlike other solutions, it enables the rapid implementation of all desired data quality functions. This includes all applications that collect or change data from customers or interested parties, without high integration or development costs. Its system neutrality and the ability to add functions flexibly and modularly at the touch of a button make the Data Quality Hub a lean, targeted solution. Data stewards can work directly in the hub interface if required.
Modular use of data quality functions
A direct view into your data
Web interface for transparent data access
In addition to the data quality functions, the Data Quality Hub offers an interface that provides quick and easy insights into your data. Data quality workflows are controlled centrally here. Dashboards and KPIs provide information on the status of data quality and the success of measures. Data can also be edited directly via a dedicated data steward interface.
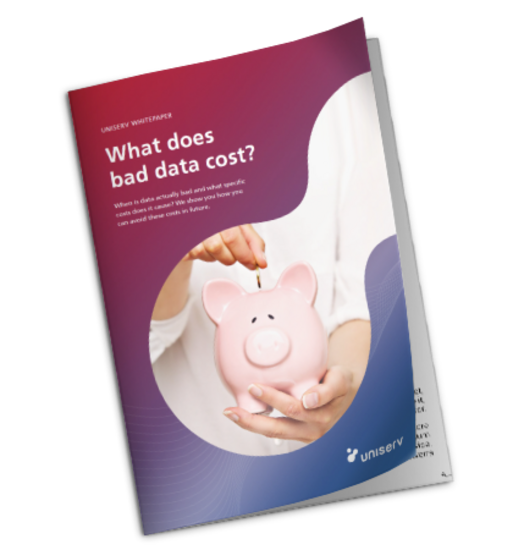
What does bad data cost?
The price for poor data quality is high. However, incorrect addresses and poor data quality are a cost factor that companies could easily avoid.
Read our paper ‘What does bad data cost?’ to find out how high such costs can be and how you can avoid them from the outset.
Good data for your business processes
The importance of data quality for your company
Prospect and customer data is at the heart of companies. They are often not treated as such. They are inaccurate, inconsistent and out of date, scattered across different systems and often can no longer be easily merged. However, specialist departments need accurate, trustworthy and relevant data, for example:
With the Data Quality Hub you get:
- relevant and valid prospect and customer data in one or more of your systems.
- the ability to create a unique customer ID and a comprehensive 360-degree view.
- fast implementation and reduced development effort.
- the flexibility to optimize and expand your data quality at any time and across systems.