Securing data quality in long term
Customer master data is only useful in the long term if its data quality is guaranteed.
Customer data is constantly changing. New information is added. People move or get married. Streets and places are renamed or added to. These changes must be constantly monitored and regularly updated, otherwise the data will eventually become unusable.
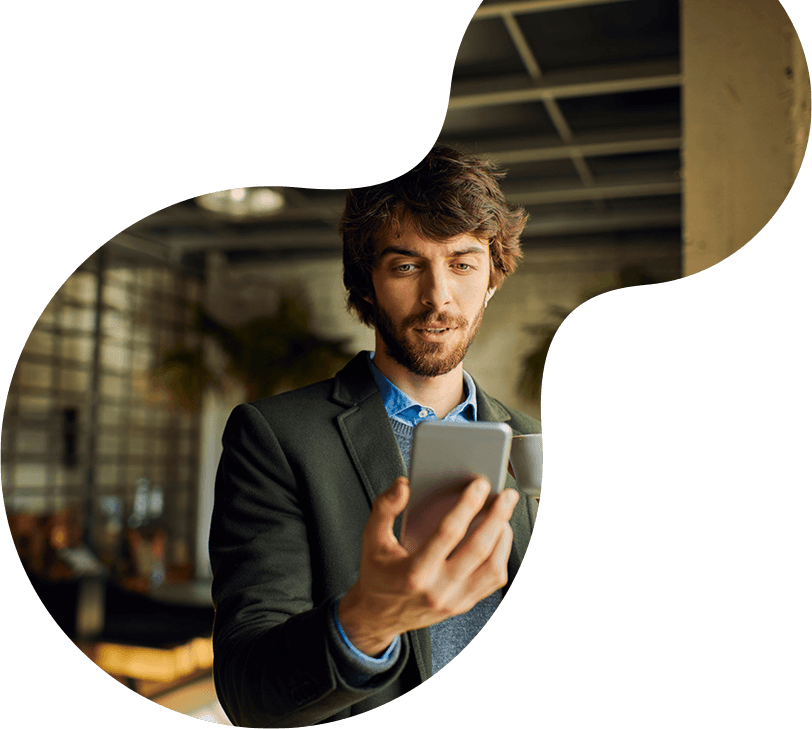
Why you need to ensure data quality in long term?
To achieve data quality, it is necessary to clean the entire database. Therefore, an initial data cleansing is carried out at the beginning of a project or initiative. However, data quality is not a “one-time action”; it is much more a long-term process. This is because customer data is subject to constant change. Therefore, on the one hand, it is important to ensure quality directly during data entry with first-time-right measures. On the other hand, anti-ageing measures must be used to prevent the ageing of customer data. Otherwise, poor data quality is likely to recur.
But where do these constant changes come from? What are the causes and causal relationships? And how do you realize that you have not got the long-term aspect of data quality assurance under control?
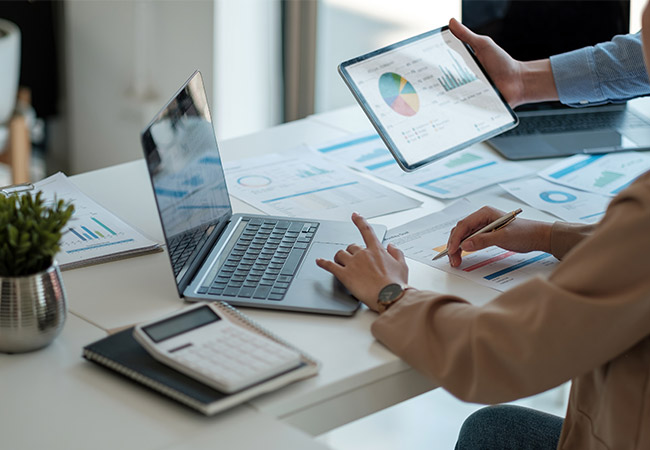
- Moving: on average, 25,000 people move house in Germany every day. However, not everyone provides information about their new address, such as banks, insurance companies and other business partners. Without an up-to-date address, however, customer contact is lost, for example for sending important mail.
- Renaming: every year, 45,000 streets and 2,000 place names change in Germany due to incorporations, renaming or new development areas. These changes must also be continuously tracked in databases, otherwise the correct address is no longer available.
- Duplicates: they are like a 'bad penny' and, despite all precautions, sooner or later they will reappear. It is therefore advisable to periodically search the entire database for duplicate and multiple records and then consolidate them so that no valuable information is lost.
- Incorrect data entry: spelling mistakes, abbreviations, typos or hearing errors – when creating or modifying customer data, errors can quickly creep in, reducing data quality and affecting processes. This must be avoided.