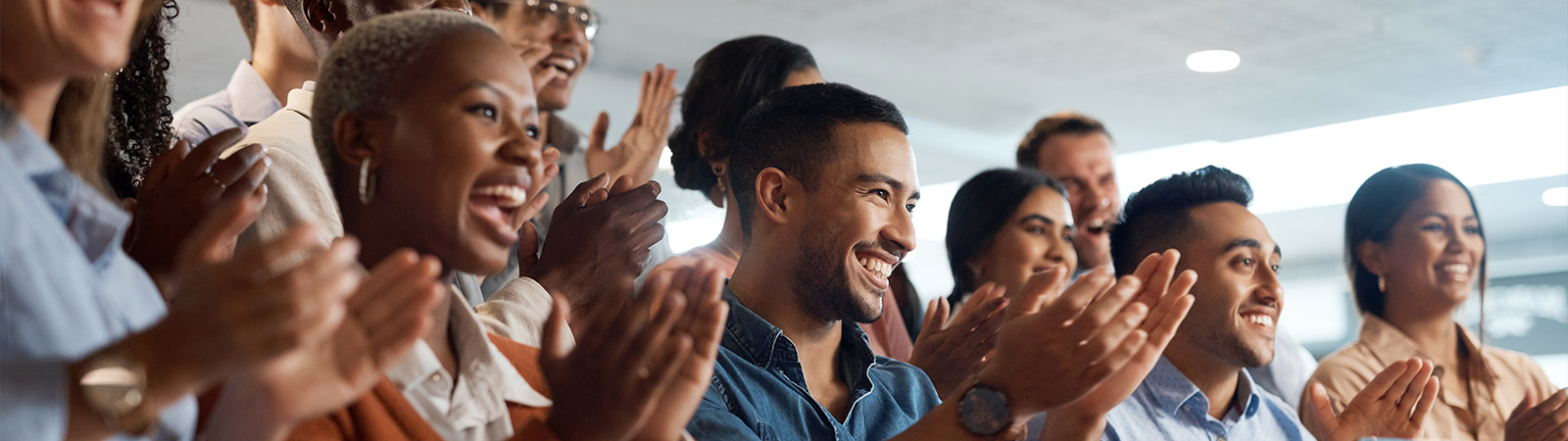
Customers
Insight. Perspective. Vision.
For us, successful project work focused on added value means working with our customers to develop and implement the optimal solution for the use case in question. This is always tailor-made and never standardized. But always based on sound experience from many projects. Get your personal insights, perspectives and visions here.
Wüstenrot & Württembergische Group.
Read our W&W Success Story to find out how 30 million master data records from different systems were unified and data turned into customer insights.
Stadtwerke Münster
Read our success story to find out how Stadtwerke Münster creates a standardized customer view by combining different sources.
Koelnmesse
In less than 6 months, Uniserv implemented a unified data view in SAP with Koelnmesse, using the Customer Data Hub and golden records.
DPD
With the Customer Data Hub, DPD validates up to 1.5 million addresses per day almost in real time, for optimized data quality with efficient processes through unique golden records.
Volkswagen Financial Services
Caution and Compliance Framework from Uniserv integrated into the SAP workflow efficiently and significantly identifies and reduces the number of actual fraud cases.
KIND Hearing Instruments
Analyzing and cleansing within a customer master data platform as part of the smooth implementation and optimization of ERP solutions.
R+V
Read how R+V improves the customer journey with the address validation location in the success story.
Suva - Swiss social insurance
Read the success story to find out how Suva improved data quality and increased efficiency by migrating to SAP S/4HANA.
LucaNet
Read how LucaNet managed to merge 600,000 scattered customer data & guarantee high data quality in the success story.